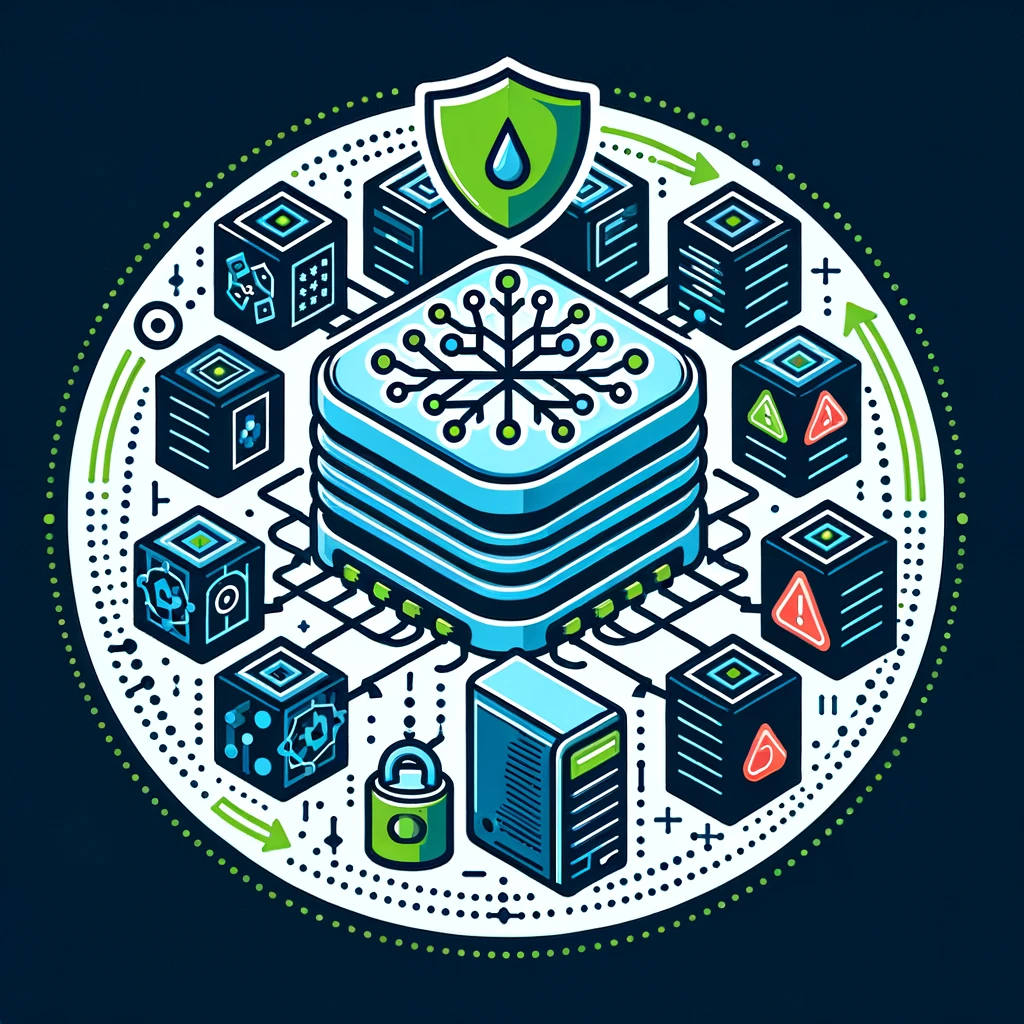
Security of Machine Learning in High-Performance Computing
Machine Learning (ML) security, especially in the context of High-Performance Computing (HPC), is a critical area of research that addresses the vulnerability of ML systems to various forms of attacks and threats. With the increasing reliance on HPC infrastructures to train complex ML models, these systems become attractive targets for adversaries looking to exploit weaknesses in computational resources, data integrity, and algorithmic trustworthiness. Potential attacks include data poisoning, where maliciously modified data is used to train models, resulting in compromised outputs; model stealing, where attackers infer the model's parameters; adversarial attacks, designed to deceive models into making incorrect predictions; and privacy attacks like Membership Inference Attacks (MIA), which threaten user privacy by deducing individual data point involvement in the training dataset. Our research is motivated by the challenge of securing ML systems within HPC environments. Our objective is to not only explore and identify these vulnerabilities but also to devise and implement robust defense mechanisms to mitigate such risks effectively, thereby advancing the reliability and trustworthiness of ML applications in critical settings.
Recent Publications
“Whispering MLaaS” Exploiting Timing Channels to Compromise User Privacy in Deep Neural Networks | CHES 2023
While recent advancements of Deep Learning (DL) in solving complex real-world tasks have spurred their popularity, the usage of privacy-rich data for their training in varied applications has made them an overly-exposed threat surface for privacy violations. Moreover, the rapid adoption of cloud-based Machine-Learning-asa-Service (MLaaS) has broadened the threat surface to various remote side-channel attacks. In this paper, for the first time, we show one such privacy violation by observing a data-dependent timing side-channel (naming this to be Class-Leakage) originating from non-constant time branching operation in a widely popular DL framework, namely PyTorch. We further escalate this timing variability to a practical inference-time attack where an adversary with user level privileges and having hard-label black-box access to an MLaaS can exploit Class-Leakage to compromise the privacy of MLaaS users. DL models have also been shown to be vulnerable to Membership Inference Attack (MIA), where the primary objective of an adversary is to deduce whether any particular data has been used while training the model. Differential Privacy (DP) has been proposed in recent literature as a popular countermeasure against MIA, where inclusivity and exclusivity of a data-point in a dataset cannot be ascertained by definition. In this paper, we also demonstrate that the existence of a data-point within the training dataset of a DL model secured with DP can still be distinguished using the identified timing side-channel. In addition, we propose an efficient countermeasure to the problem by introducing constant-time branching operation that alleviates the Class-Leakage. We validate the approach using five pre-trained DL models trained on two standard benchmarking image classification datasets, CIFAR-10 and CIFAR-100, over two different computing environments having Intel Xeon and Intel i7 processors.
Read PaperResearch Group Members
Prof. Debdeep Mukhopadhyay
Professor, Computer Science and Engineering Department, IIT Kharagpur
Visit WebsiteProf. Pabitra Mitra
Professor, Computer Science and Engineering Department, IIT Kharagpur
Visit WebsiteDr. Sarani Bhattacharya
Assistant Professor, Computer Science and Engineering Department, IIT Kharagpur
Visit WebsiteShubhi Shukla
Research Scholar, Centre for Computational and Data Sciences, IIT Kharagpur
Visit Website